Static Spatial Models
The term “spatial model” refers to a wide family of approaches able to consider the topological, geometric, or geographic characteristics of entities during the evaluation. This kind of models is often combined with GIS for data input, analysis and/or display. The transition from non-spatial to spatial model is extremely significant in assessing urban and territorial transformation and planning. The research group experiments the integration of GIS potentialities into more consolidated evaluation techniques, such as multicriteria analysis and hedonic price model, or more innovative ones, such as agent-based model and system dynamics model.
Multi-criteria spatial decision support system (MC-SDSS)
In the context of spatial complex problems, Multicriteria-Spatial Decision Support Systems (MC-SDSS) (Malczewski, 1999) are becoming increasingly relevant and applied to different fields (Malczewski, 2006)(Ferretti, 2012): from territorial to urban planning from waste management to environmental protection, from hospital site selection to assessing urban energy scenarios.
From a methodological point of view, MC-SDSS is an interactive computer system able to assist the user, or a group of users, to achieve high level of effectiveness in decision-making process (Malczewski, 1999).
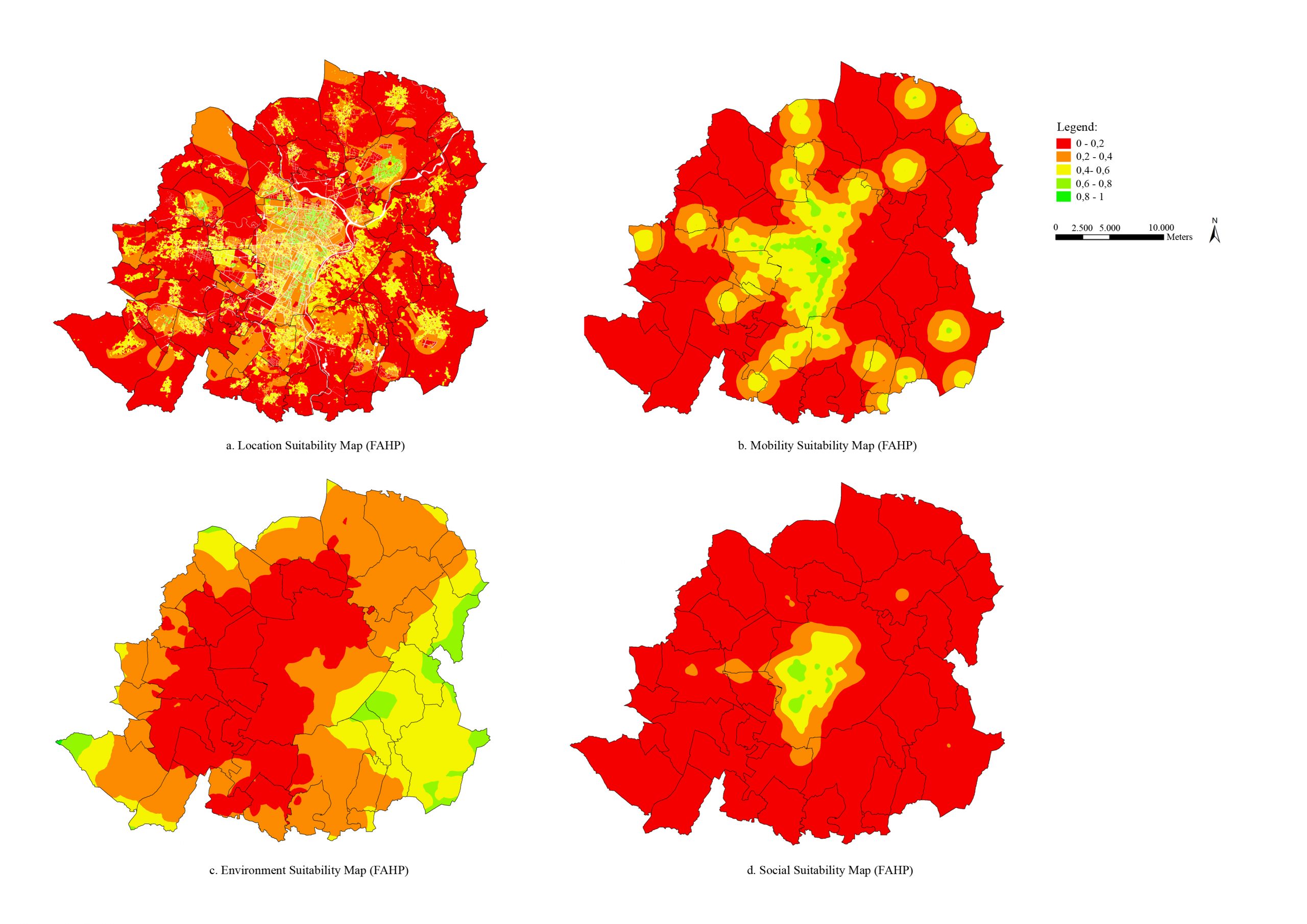
The problems able to solve are called by Simon (Simon, 1960) as semi-structured spatial decision problem, where the solution results from the contribution of decision-makers and computer tools.
MC-SDSS are characterized by the combination of Geographic Information Systems (GIS) and Multicriteria Decision Analysis (MCDA) to support users in spatial decision processes, thanks to the generation of geographical alternatives which respect a set of criteria (Jelokhani-Niaraki & Malczewski, 2015a). The combination of geographic data (criteria maps) and Decision Maker’s preferences and uncertainties (value judgement) provides information for decision-making and for an overall assessment of alternatives (Comino, Bottero, Pomarico, & Rosso, 2014).
Compared with traditional methods, MC-SDSS integrates the potential of GIS and MCDA in a combined manner, introducing an explicit geographical component in the evaluation (Ruiz, Romero, Pérez, & Fernández, 2012). On one hand, GIS is a powerful set of tools for collecting, storing, retrieving, transforming and displaying spatial data (Burrough & McDonnell, 1986). The possibility to visualize data through maps can facilitate stakeholders’ debate and can solve conflicts among them. In addition, the display of data can increase the number of parties called to the discussion, thanks to a visual language that is easy to explain and understand (Haertsch & Smith, 2012). On the other hand, MCDA enables to consider all the aspects that contribute to the identification of proper alternatives and to compare them with weights and value judgement. MCDA procedures help to build a consensus across the decision alternatives through mechanisms of revealing preferences and facilitate approval of solutions shared by all stakeholders (Jelokhani-Niaraki & Malczewski, 2015b).
BOTTERO, M., COMINO, E., DURIAVIG, M., FERRETTI, V., & POMARICO, S. (2013). The application of a Multicriteria Spatial Decision Support System (MCSDSS) for the assessment of biodiversity conservation in the Province of Varese (Italy). Land Use Policy, 30(1), 730-738.
OPPIO, A., BOTTERO, M., FERRETTI, V., FRATESI, U., PONZINI, D., & PRACCHI, V. (2015). Giving space to multicriteria analysis for complex cultural heritage systems: The case of the castles in Valle D’Aosta Region, Italy. Journal of Cultural Heritage, 16(6), 779-789.
COMINO, E., BOTTERO, M., POMARICO, S., & ROSSO, M. (2014). Exploring the environmental value of ecosystem services for a river basin through a spatial multicriteria analysis. Land Use Policy.
Spatial Econometric Model
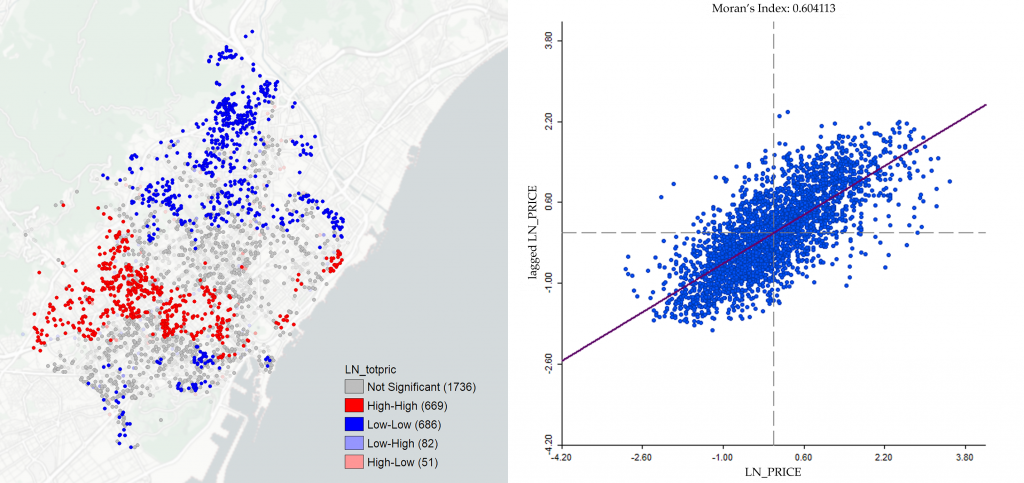
When dealing with spatial data through hedonic price models, it can happen that the variables are characterized by spatial dependence (Anselin et al. 2008; LeSage and Pace 2008). In the real estate market, spatial autocorrelation is mainly due to the characteristics of the urban fabric and to the distribution of properties with similar intrinsic and extrinsic characteristics that can affect market prices. The formation of offer prices by real estate agents can cause an autocorrelation effect (Ismail 2006). Ordinary Least Square models are inefficient in similar cases.
The scientific literature mainly recognizes two models for correcting spatial autocorrelation in hedonic regression models. The Spatial Autoregressive (SAR) model assume that the dependence exists directly between the values of the dependency variable, which in the case of studies in the field is represented by the price of the properties. The SAR models predict that a spill over effect exists in the real estate market, and that the prices of neighbouring transactions manage to influence the formation of the price for the object under investigation. A second model, named Spatial Error Model (SEM), evaluates the extent to which spatially distributed variables not considered in the model can influence the dependent variable (Anselin and Lozano-Gracia 2008).
The VALIUM group has established connections with international research institutes in this research area. In particular, the collaboration with the Center de Política de Sòl i Valoracions (CPSV) of the Universitat Politècnica de Catalunya (UPC) in Barcelona led to the publication of “EPC Green Premium in Two Different European Climate Zones: A Comparative Study between Barcelona and Turin” (Dell’Anna et al. 2019). In this paper, the green premium deriving from the Energy Performance Certification (EPC) was investigated by proposing a comparison between the city of Barcelona and Turin using spatial hedonic model. With reference to the most significant publications, “Valuing buildings energy efficiency through Hedonic Prices Method: are spatial effects relevant?” we apply spatial models connected to the theory of hedonic prices to estimate the implicit marginal price, as a measure of the willingness to pay for the energy consumption of buildings in the city of Turin (Bottero et al. 2018).
BOTTERO, M., BRAVI, M., MONDINI, G., TALARICO, A. (2016). Buildings Energy Performance and Real Estate Market Value: An Application of the Spatial Auto Regressive (SAR) Model. In: Stanghellini S., Morano P., Bottero M., Oppio A. (eds) Appraisal: From Theory to Practice. Green Energy and Technology. pp. 221–230. Springer, Cham.
BOTTERO, M., BRAVI, M., DELL’ANNA, F., MONDINI, G. (2018). Valuing building energy efficiency through Hedonic Prices Method: are spatial effects relevant?. Valori e Valutazioni, 21, 27-40
DELL’ANNA, F., BRAVI, M., MARMOLEJO-DUARTE, C., BOTTERO, M.C., CHEN, A., (2019). EPC green premium in two different European climate zones: A comparative study between Barcelona and Turin. Sustainability, 11, 5605.
Spatial Dynamics Models
Dynamic models refer to a class of approaches where system properties change over time, through the development of equations or computer code. The main difference of dynamic models over static models is mainly on the time-dependence in the state of the system. The research group is involved in the study of the pros and cons of different dynamic models (such as agent-based model/multi-agent system, system dynamics model and Lokta-Volterra model) in supporting urban and territorial assessment and development.
Agent-Based Model (ABM)/ Multi-agent system (MAS)
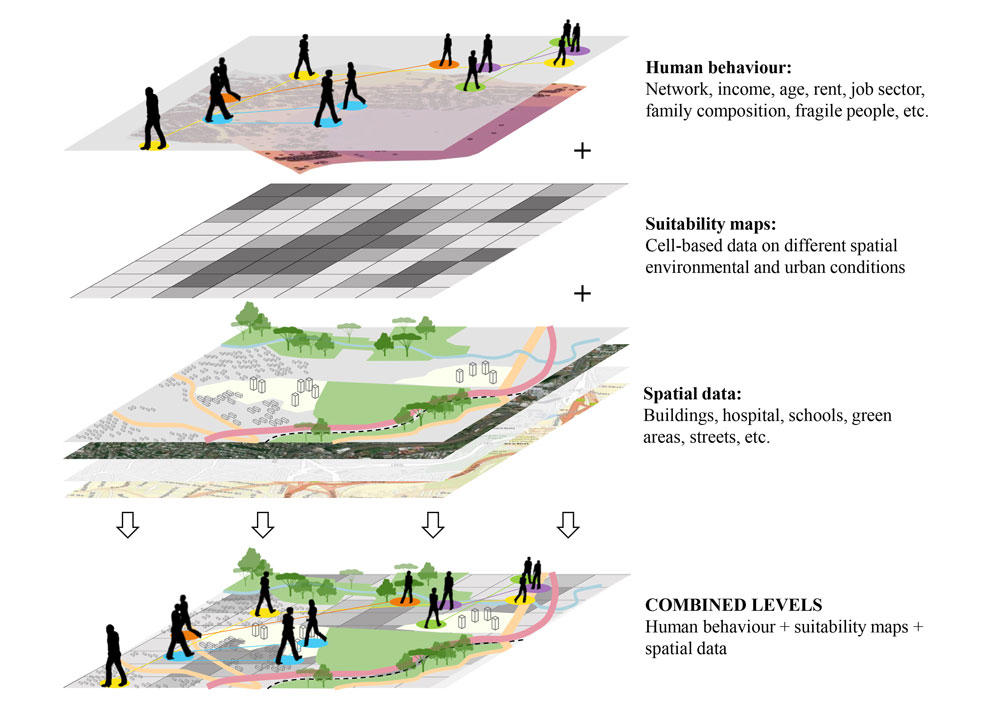
Agent-based modeling (ABM) or multi-agent system (MAS) has become a widely used approach in many disciplines in the last 15 years (Heppenstall et al.2012), spanning physic, biology, ecology, social sciences, economy and geography (Macal and North 2014; Grimm et al.2006).The increasing development of ABM/MAS goes in parallel with the study of complex systems, characterized by non-linear relationships between constantly changing entities (Parker et al.2003).These models seem to be particularly successful in these studies, mainly because they rely on the idea of effects and synergetic characteristics emerging from the analysis of subcomponent relationships (Parker et al.2003).
In fact, ABM/MAS allows to study and verify the influence of decisions at the micro level which effects emerging at macro level (Crooks et al.2008). Agents are the crucial component in this model. There is no universal agreement among researchers about the precise definition of agent (Macal and North 2014), beyond the essential property of autonomy (Parker et al.2003). The autonomy characteristic means that agents have control over their actions and internal state in order to achieve goals. Agents are individual objective-oriented entities, which interact with the others inside of a shared environment. This environment can provide only information about the spatial location of each agent, but also a rich set of information and data, which are available for the agent through a GIS integration (Parker et al. 2003). The “exploratory simulation” capability of ABM/MAS is fundamental in studying complex system, as the urban one.
The research group adopts ABM/MAS to test different sustainable policies at the district scale, verifying the effectiveness and efficiency based on the analysis of the behaviours and decision rules of the inhabitants, as well as to predict, before the implementation, the multiple effects and outcomes generating by urban transformations in consolidated areas. The development of an ABM, strongly based on a bottom-up approach in the evaluation of different strategies and scenarios, could be an opportunity to create an alternative perspective of these complex problems, thus supporting decision-makers and planners in the definition of policies and strategies for urban development. Moreover, our applications are able to identify the key drivers and key effects which guide these processes and connect them to the specificities of a place and its population.
CAPRIOLI, C., BOTTERO, M.C. Agent-based modelling and Geographic Information System for the evaluation of eco-district’s scenarios. Proceedings of the Environmental and territorial modelling for planning and design; Leone, A., Gargiulo, C., Eds.; FedOAPress: Naples, 2018; pp. 35–45.
CAPRIOLI, C., BOTTERO, M., PELLEGRINI, M. (2019). An Agent-Based Model (ABM) for the Evaluation of Energy Redevelopment Interventions at District Scale: An Application for the San Salvario Neighborhood in Turin (Italy). M. S. et Al. (Ed.), Computational Science and Its Applications – ICCSA 2019. ICCSA 2019. Lecture Notes in Computer Science, vol 11621 (pp. 388–403).
System Dynamics Model for urban resilience assessment
Cities are actually exposed to a wide range of stresses and shocks, both natural and man-made. These perturbations include all city dimensions from the environmental to the social and economic. To face with these conditions, the global community is increasingly that building urban resilience is needed to make cities able to adapt, change, and respond to this high level of uncertainty.
In the context of urban resilience enhancement, new evaluation methods that are able to predict the possible effects of urban policies and programs are needed to support the Decision Making process. Related to this challenge, System Dynamics Models (SDMs) can be proposed as a method to support the definition of policies to enhance urban resilience. SDMs are both theoretical and computer-aided approach that are applied to describe and simulate the dynamic behaviour of complex systems. Both for their ability to consider the mutual interrelation among the variables of complex systems and their capacity to predict the possible evolution of complex systems over time using a set of Ordinary Differential Equations(ODEs), SDM are actually used as Decision Support System in urban transformation field.
In this sense, the main objectives of applying SDM in urban resilience assessment are:
- Providing a decision support system able to predict both the effects and impacts of policies and programmes on urban system in terms of urban resilience enhancement (Ex-ante evaluation);
- Proposing an integrated evaluation approach based on SDM and Multi-Criteria Analysis (MCA) in order to evaluate urban resilience both in its multidimensionality and dynamicity over time. The final aim is to obtain final synthetic indices to compare initial condition with the future state of the urban systems in term of urban resilience performance
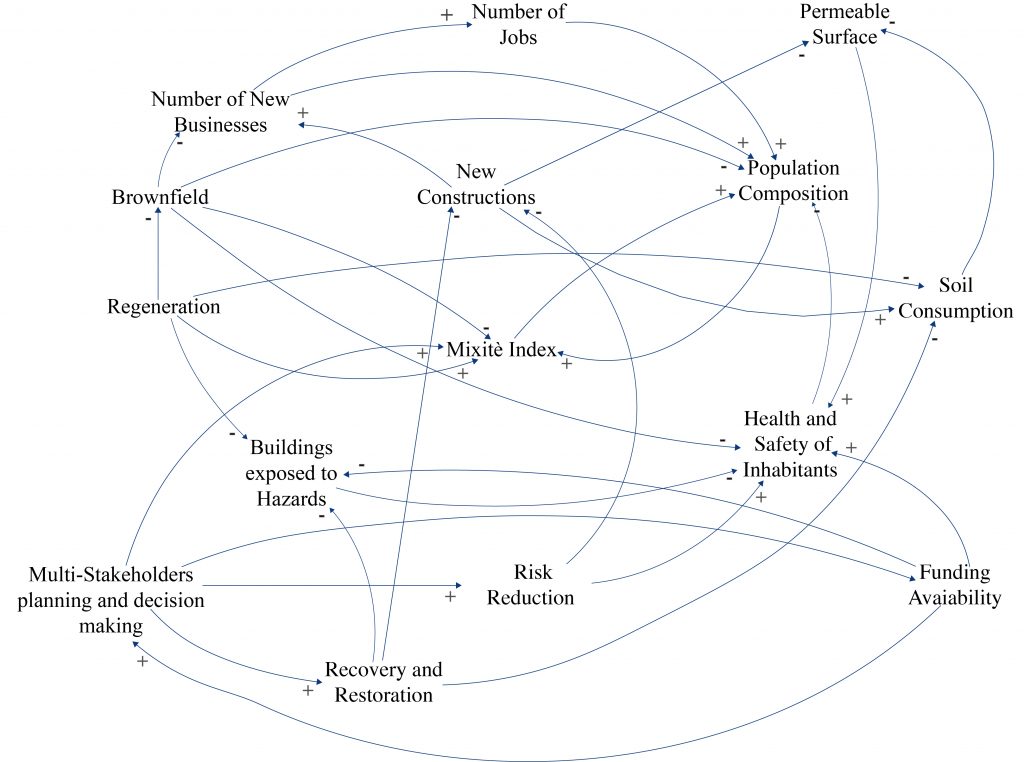
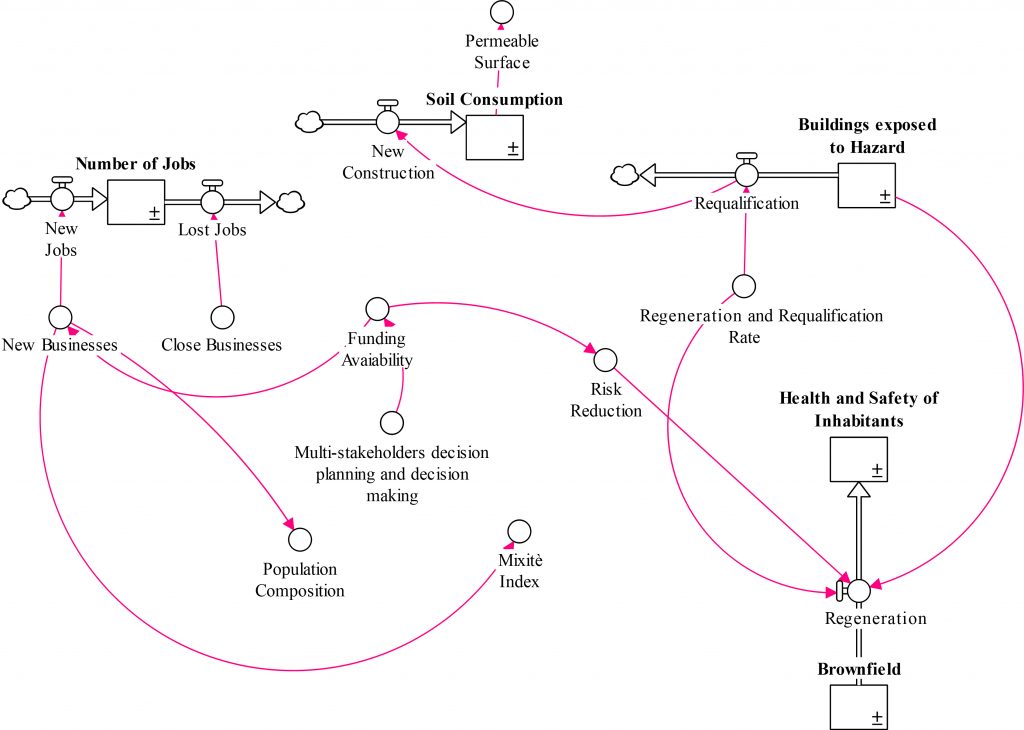
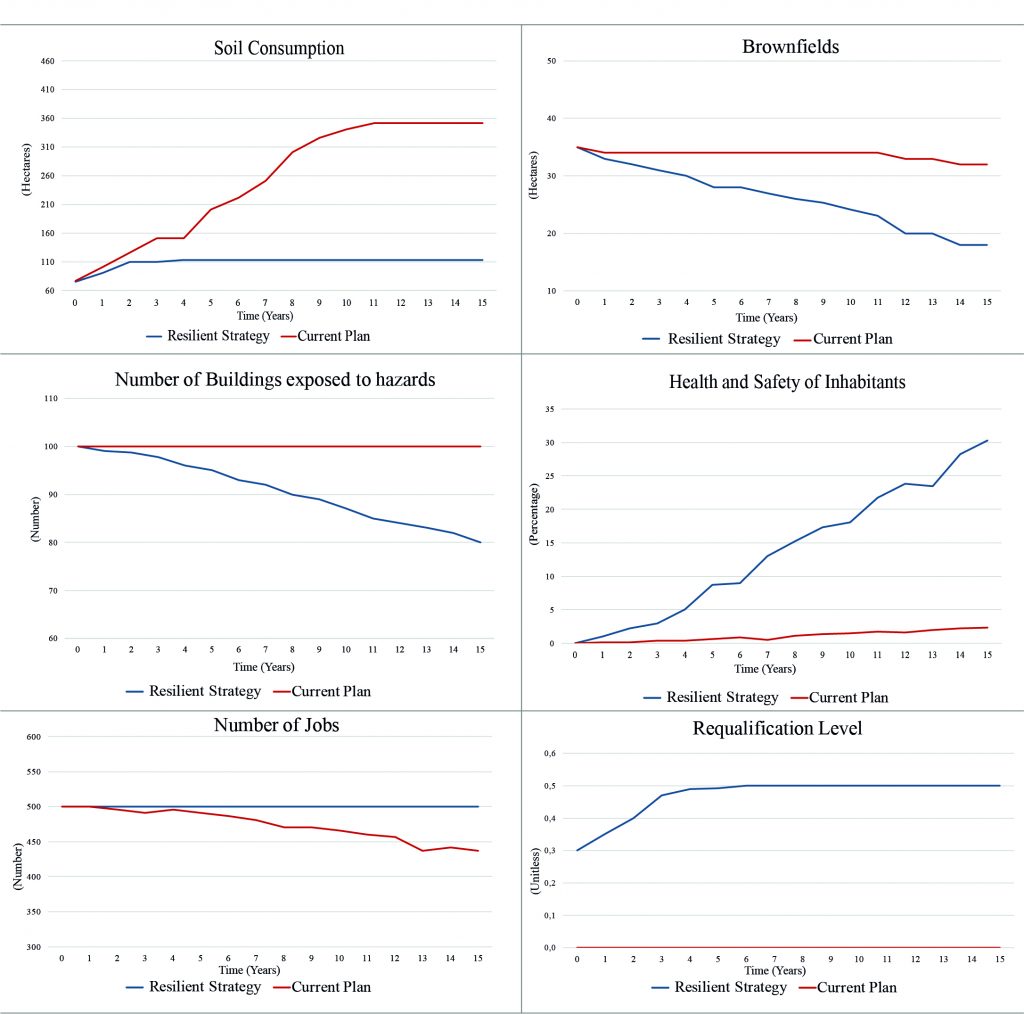
ASSUMMA, V., BOTTERO, M., DATOLA G., DE ANGELIS, E., MONACO, R. (2020) Dynamic Models for Exploring the Resilience in Territorial Scenarios. Sustainability.
DATOLA G., BOTTERO M., DE ANGELIS E. (2019) How Urban Resilience Can Change Cities: A System Dynamics Model Approach. Misra S. et al. (eds) Computational Science and Its Applications – ICCSA 2019. ICCSA 2019. Lecture Notes in Computer Science, vol 11622. Springer, Cham.
Lotka Volterra model
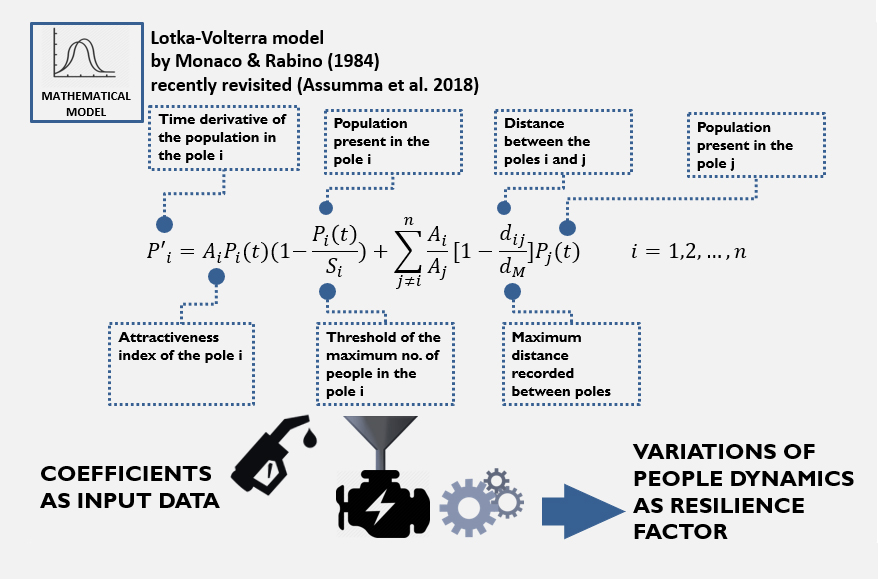
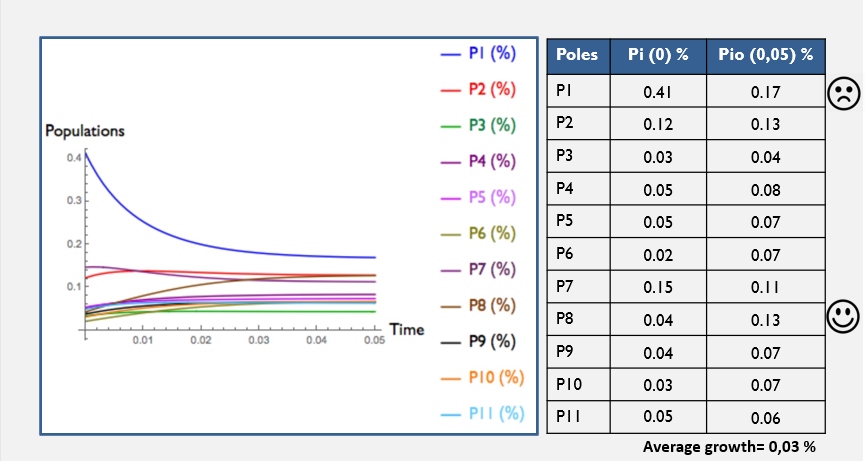
Mathematical modelling provides reliable models for investigating the dynamics of complex systems dynamics and predicting future possible scenarios of long-time regime. The Lotka-Volterra models of cooperative type named PANDORA and the network models are able to trigger useful insights for cities and territories in the field of environmental monitoring and assessment (Monaco and Soares, 2017; Bonacini et al. 2018), thanks to their ability to integrate different disciplines, such as mobility (Monaco and Rabino, 1984), landscape ecology (Murray 2002; Gardner and Turner 2015), GIS methods (Malczewski, 2006) land use planning (Gobattoni et al. 2011), economics (Assumma et al. 2018, 2019), land take evaluation (Monaco and Servente 2006) and so on.
Over the last years, these dynamic models reveal particular useful to explore urban and territorial resilience as part of wide evaluation framework to aid the decision makers in better interpreting cities and territories dynamics and defining suitable policies and actions to increase their resilience with respect to the ongoing climate change context (Elmqvist et al. 2019).
ASSUMMA, V., BOTTERO, M., DATOLA, G., DE ANGELIS, E., MONACO, R. (2020). Dynamic Models for Exploring the Resilience in Territorial Scenarios. Sustainability, Vol 12, pp. 1-19.
ASSUMMA, V., BOTTERO, M., MONACO, R., SOARES, AJ. (2019). An integrated evaluation methodology to measure ecological and economic states of an environmental system: an application in Piedmont (Italy). Ecological Indicators, vol 105, pp 156-165.
ASSUMMA, V., BOTTERO, M., MONACO, R., SOARES, A.J. (2018). An Integrated evaluation model for shaping future resilient scenarios in multi-pole territorial systems. Environmental and territorial modelling for planning and design, pp. 52-55.